ABIDI
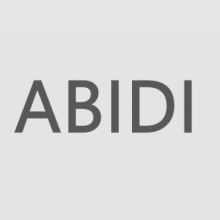
El proyecto ABIDI con referencia PCI2019-103762, ha sido financiado por el Ministerio de Ciencia, Innovación y Universidades, en la Convocatoria Programación conjunta internacional 2019-Programa Estatal de I+D+I Orientada a los Retos de la Sociedad, en el marco del Plan Estatal de Investigación Científica y Técnica y de Innovación 2017-2020.
CHIST-ERA - Call Topic: Big data and process modelling for smart industry (BDSI), Call 2017
ABIDI addresses key challenges of reliable industrial IoT networks, big data analytics, edge computing, contextualization, veracity and knowledge discovery, which are fundamental issues for industrial IoT and big data analytics to be successful in industry. The project carries out fundamental research and the technical results will support a more principled transition towards fully automated processes realized by autonomous and context-aware devices. Moreover, ABIDI applies the technical results in the context of realistic industry use cases and performs systematic studies with professional experts about the usability and reliability of the produced technologies.
Industry’s transition to the digital world has already reaped benefits such as fully automated processes and cost savings and has paved the way for novel services and business models.
However, the challenges of data-driven autonomous and context-aware systems that enable the automated processes in industry have not been fully addressed. ABIDI project contributes to the automated industrial processes with the development of a framework for context-aware and veracious big data analytics with automated knowledge discovery and reasoning for industrial IoT. Our goals are building reliable industrial IoT networks, automating the processing of big variety, volume, and velocity industrial IoT data streams, and offering big data insights with centralized or distributed solutions. Therefore, we develop adaptive models-based decision support and recommendation tools that enable the automated control of the system with processing big industrial IoT data. To materialize the research results into innovations that industrial operators and service providers can utilize, we design four real-life use cases with respect to energy consumption prediction, including two academic use cases in CeDInt-UPM (Spain) and HES-SO (Switzerland), and two industry use cases with Oulun Energia (Finland) and Debiopharm (Switzerland). Energy efficiency is one of the KPIs for any industry regardless of line of business.
The vision of digitalization and fully automated processes realized by autonomous and context-aware devices is taking hold in industry. Several terms with slightly different areas of emphasis have been utilized for this vision, including Internet of Things (IoT), Industrial Internet, Industrial Internet of Things (IIoT) and Industry 4.0. Kevin Ashton, the coiner of the term IoT, elaborates the fundamental idea behind the general vision as follows:
“If we had computers that knew everything there was to know about things - using data they gathered without any help from us - we would be able to track and count everything, and greatly reduce waste, loss and cost. We would know when things needed replacing, repairing or recalling, and whether they were fresh or past their best.”
A huge amount of research has been executed to realize the IIoT vision, but a lot of work is still required, especially on reliable IIoT networks, big data analytics, contextualization, veracity, and knowledge discovery. Data is the new oil of digital economy and we need to empower IIoT systems with means 1) to reliably collect big data from heterogeneous and unpredictable sources in complex IIoT systems; 2) to enrich the data with context and veracity metadata; 3) to produce prediction models-based decision support tools for data utilization; 4) to break silos and foster information interoperability by adding semantic metadata; and 5) to derive trustful, reliable, and latency-sensitive system-level understanding and knowledge for decision making.
To achieve these goals, we propose a framework for context-aware and veracious big data analytics with automated knowledge discovery and reasoning for industrial IoT. The development of this framework addresses the following main challenges:
Reliable Industrial IIoT networks: to develop novel network mechanisms that work on the IEEE 802.15.4-2015 definition and to support high reliable IP-based communications to achieve bounded and low delays.
Optimized IIoT Network Architecture: to define and implement a network architecture consisting of a set of mechanisms and protocols for the different communication layers that enables the integration of new IIoT devices and assures interoperability with existing systems at the industrial premises.
Edge computing for IIoT: to move knowledge-centric computations from the central big data infrastructure to the edges of the IIoT systems. Edge nodes also provide mechanisms for adding context and veracity metadata and for handling knowledge synchronization between the big data infrastructure and edge devices.
Big data processing: to identify and tune the most suitable off-the-shelf big data solutions to improve performance when considering the characteristics IIoT and edge computing.
Balancing the computation between Big Data infrastructure and edge devices: to balance the workload between resource limited and contextual edge devices and the powerful central big data infrastructure.
Adaptive data processing for veracious big data streams: to develop mechanisms for improving the veracity of sensor data that will be fed to adaptive prediction models. To utilize semantic context data for intelligent integration of the data streams with relevant background knowledge for IIoT services.
Adaptive prediction models and automatically processed veracious data streams with big variety, volume, and velocity: to develop prediction models that provide information for the decision making tools. To improve the performance of the decision making tools with adaptive prediction models that can learn from streaming data.